We curated a lot about Artificial Intelligence (AI) key market insights, new technology developments and the investment done in that area at AthisNews. This is the hot topic well-adopted by experts and still on his way by the society. After reading couple of studies this week, we decided to give you a deeper overview to get the basics and also deeper market insights regarding this field. “Artificial Intelligence” is a series of guides to understand what is this technology (part 1), when this technology meets real businesses and offers new ways to do jobs (part 2), what are the worldwide AI tech actors (Part 3) and what are the commercially available core technologies & frameworks you can find in the market (Part 4).
What is Artificial Intelligence?
Let’s start with some short definitions and historical stuff! Well, Artificial intelligence (AI) means getting a computer to mimic human behaviour in some way. The goal then, as now, was to get computers to perform tasks as human: things that required intelligence. Initially, researchers worked on solving logic problems like playing checkers. The computer is doing something intelligent, so it’s exhibiting intelligence that is artificial.
You said history? First of all, in 1950, the computer scientist Alan Turing proposed a test for machine intelligence. If a machine can trick humans into thinking it is human, so then it has intelligence.
“Every aspect of learning or any other feature of intelligence can in principle be so precisely described that a machine can be made to simulate it.”
– John McCarthy in august 31, 1955, at the proposal for the Dartmouth summer research project on artificial intelligence.
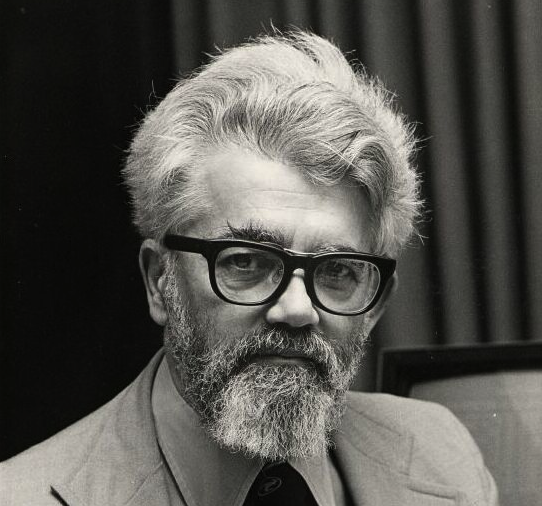
John McCarthy who is the Computer scientist known as the “father of AI”. Indeed, he played a significant role in defining the development of intelligent machines. The term “cognitive scientist” was originally coined in his 1955 proposal for the 1956 Dartmouth Conference, the first artificial intelligence conference. They brought the preliminary blocks to define ways to build a machine that could reason like a human with abstract-thought, problem-solving and self-improvement capabilities. In 1958, he created the Lisp computer language, which became the standard AI programming language.
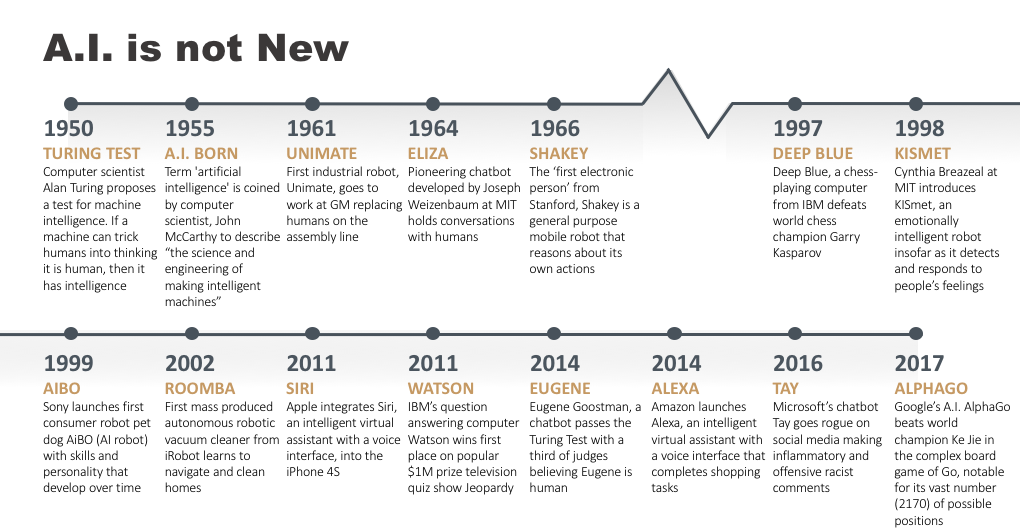
There are many different techniques to solve problems, one category of techniques started becoming more widely used in the 1980s: machine learning.
You said machine learning?
Yes, this is a subset of AI, and it consists of the techniques that enable computers to figure things out from the data and deliver AI applications. Those statistical techniques give to the machine the ability to “learn” and get “experience”. Learn you said? According to Christoph Bishop, this is more accurate to say that the machine progressively improves performance on a specific task from data, without being explicitly programmed. Indeed, as a human, the core objective of a learner is to generalize from its experience. In this context, generalization is the ability of a learning machine to perform accurately on new, unseen examples/tasks after having experienced a learning data set. There is plenty of algorithm approaches to reach this objective.
“Pattern recognition has its origins in engineering, whereas machine learning grew out of computer science. However, these activities can be viewed as two facets of the same field, and together they have undergone substantial development over the past ten years.”
– According to Christopher Bishop, Technical Fellow and Laboratory Director, Microsoft Research Cambridge.
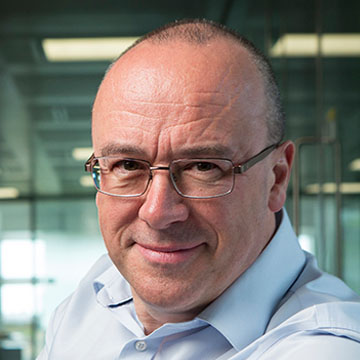
About the history behind? The name machine learning was coined in 1959 by Arthur Samuel. Machine learning explores the study and construction of algorithms that can learn from and make predictions on data – such algorithms overcome following strictly static program instructions by making data-driven predictions or decisions, through building a model from sample inputs.
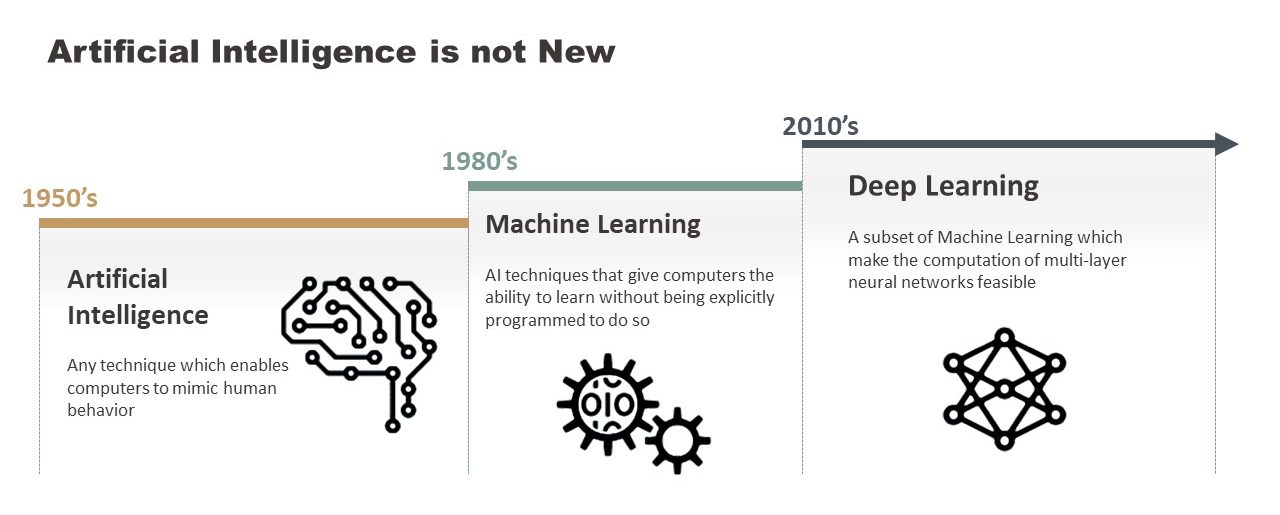
You said plenty of algorithm approaches to machine learning?
Yes, there are more than 50 different types of approaches to create learning for the computer. Those algorithms, meanwhile, are subset of machine learning that enables computers to solve more complex problems. Artificial Neuron Network, Bayesian networks and Decision tree learning are the most popular ones.
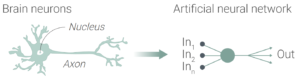
An Artificial Neuron Network (ANN) – popularly known as Neural Network is a computational model based on the structure and functions of biological neural networks. It is like an artificial human nervous system for receiving, processing, and transmitting information in terms of Computer Science.

Decision tree learning – Decision tree learning uses a decision tree as a predictive model, which maps observations about an item to conclusions about the item’s target value. What is a decision tree? I am pretty sure that you use that every day on your life! Let’s forget the formal definition and think conceptually about Decision trees. Imagine you’re sitting in your office and feeling hungry. You want to go out and eat, but lunch starts at 1 PM. What do you do? Of course, you look at the time and then decide if you can go out. You can think of your logic like this:
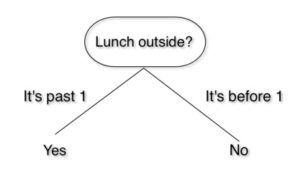
This is a simple one, but we can build a complicated one by including more factors like weather, cost, etc…
Bayesian networks are a type of probabilistic graphical model that uses Bayesian inference for probability computations. Bayesian networks aim to model conditional dependence, and therefore causation, by representing conditional dependence by edges in a directed graph. Through these relationships, one can efficiently conduct inference on the random variables in the graph through the use of factors.
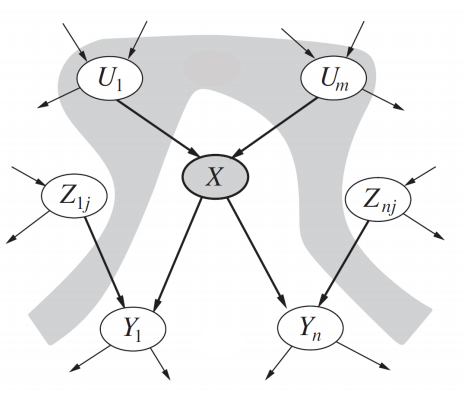
What about Deep learning? Is that different from other approaches? Deep learning is all about using neural networks with more neurons, layers, and inter-connectivity. This type of machine learning is capable of adapting itself to new data and training its systems to learn on their own and recognize patterns. We’re still a long way off from mimicking the human brain in all its complexity, but we’re moving in that direction. It started to be used mostly in hardware and in the development of GPUs for personal use in the last few years which have contributed to the development of the concept of deep learning.
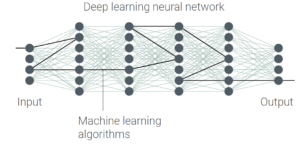
This approach tries to model the way the human brain processes light and sound into vision and hearing. Some successful applications of deep learning are computer vision and speech recognition. And when you read about advances in computing from autonomous cars to Go-playing supercomputers to speech recognition, that’s deep learning under the covers. You experience some form of artificial intelligence. Behind the scenes, that AI is powered by some form of deep learning.
Let’s look at a couple of problems to see how deep learning is different from simpler neural networks or other forms of machine learning… If I give you images of horses, you recognise them as horses, even if you’ve never seen that image before. And it doesn’t matter if the horse is lying on a sofa, or dressed up for Halloween as a hippo. You can recognise a horse because you know about the various elements that define a horse: shape of its muzzle, number and placement of legs, and so on.
Deep learning can do this. And it’s important for many things including autonomous vehicles. Before a car can determine its next action, it needs to know what’s around it. It must be able to recognise people, bikes, other vehicles, road signs, and more. And do so in challenging visual circumstances. Standard machine learning techniques can’t do that.
Stay tuned, “Artificial Intelligence” is a series of guides to understand what is this technology. Let wait for the chapter 2 “when this technology meets real businesses and offers new ways to do jobs”.
Emerging Techs addict, Thomas is specialised on Nano/Micro Techs & Semiconductors Market. Thomas holds a PhD in Microelectronics for wireless & imaging applications & a Master Degree in Sales & Marketing. He has 13+ years of demonstrated achievement in managing projects & Technology Developments. At AthisNews, he shares fresh Market Insights & Technology Analysis done by global experts.
As an Expert in IT & AI, Mickael brings fresh news about Emerging, Wearable Techs, and IT Innovation. He has 12+ years as a Software Engineer in IT and Telecom companies He is now contributor at Athis News.